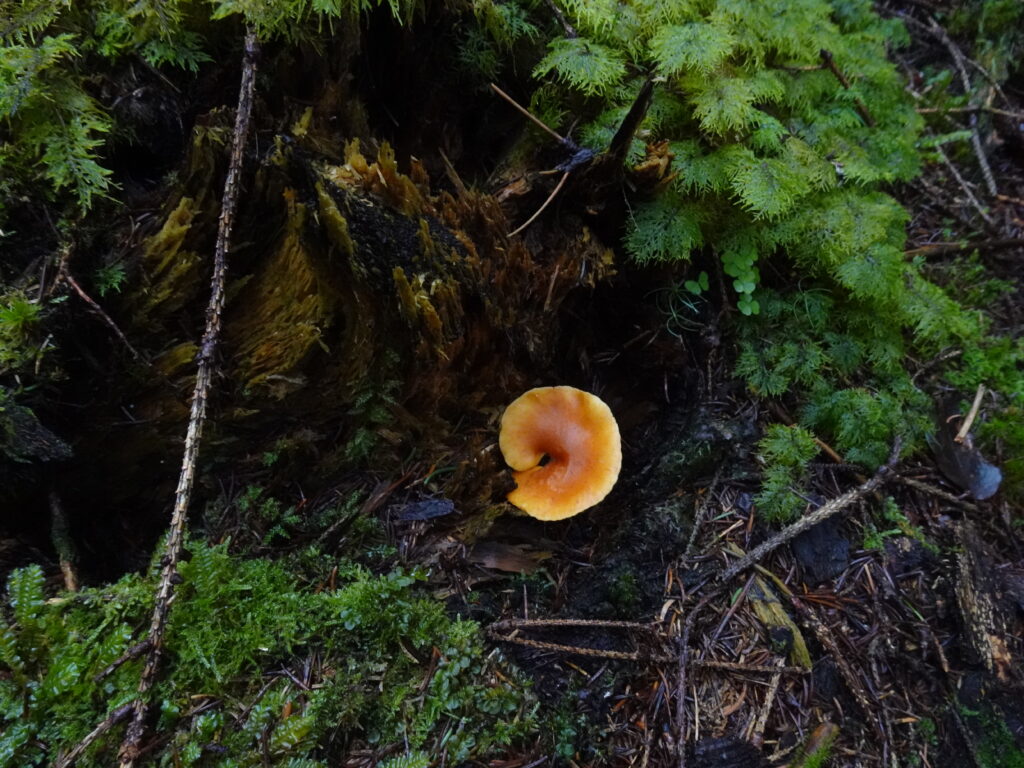
This is a course note and a documentation of the 4 day workshop at the Interface Culture, Kunstuniversität Linz, Austria. The course takes place from January 26 – 29, 10-17:00 Online. The course is given only to the registered students at the Kunstuniversität Linz.
AI and Machine Learning is any longer the technology that belongs to the world of Science Fiction but the technique that is available for artists and designers to explore and play. Although, it often still talked as a blackbox high-tech magic, the basic logic behind is something understandable for many of us, including the ones who are not mathematicians or computer scientists. In this course, I (who is not particularly good at math nor computer programming) try to explain you how Machine Learning works from my own journey into understanding ML. I will also show you how it could be used with e-textile sensors using open source library (ml-lib) with Pure Data. Machine learning is not a magic, but a powerful tool when capturing human movements and postures with e-textiles.
The course introduces how to make bend/pressure sensor using eeonyx resistive fabric (due to the online course limitation, we make kinesiology tape design version) , how you can read them with Arduino, how you can design user interaction by sensor placements, and how you can apply Machine Learning to multiple sensor inputs.
All the code examples used in the workshop is posted here >> https://github.com/mikst/IC_workshop
Day 1:
– Introduction Lecture
– Installing Pure Data and ml-lib, cyclon
– How Artificial Neural Network work
– Group calculation: ANN and backpropagation
– Trying out ml.ann on Pure Data
Installing Pure Data and ml-lib, cyclone
Install Pure Data Vanilla from here >> http://puredata.info/downloads/pure-data
After installing, open the Pure data, go to Help/Find externals. It will open a new window. Type ml-lib. It should find the correct externals. let it download it.
It will be also handy to have cyclone externals, which include a lot of object similar to max/msp objects. type cyclone on externals manager and download.
Afterwards, go to preferences and set the path to the externals you have just downloaded. it should be in your document/PD/externals folder.
After setting the path, open a new patch, make a new object and type in “ml.ann” and “gate”. If you see the object box with straight line (not dotted line) then your installation of externals went well. If not, check the path or restart the PD to see if it can find the externals you have just downloaded.
How Artificial Neural Network work
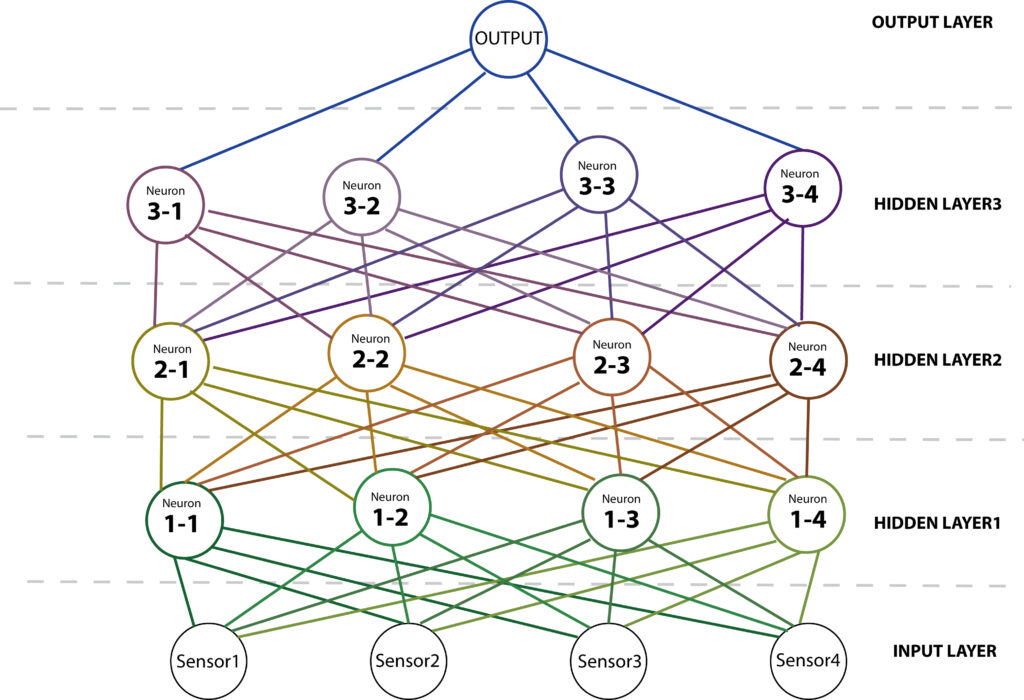
You are one of the Neurons. Make sure you know which neuron you are, and note it.
Now, assign 4 random weights (-3 to 3) to each of your connections and a bias (-3 to 3) for your neuron. write it down
layer 1: calculate the sum of your inputs:
sum = input1 * weight1 + input2 * weight2 + input3 * weight 3 + input4 * weight 4 + bias
Input your sum to sigmoid.
sigmoid(sum) = 1.0 / (1.0 + exp (-1.0 * sum));
You can use online calculator like this one
sigmoid calculator >> https://keisan.casio.com/exec/system/15157249643325
pass your output to the layer 2 neurons. your output is their input. Layer 2 neuron calculates the same process > pass the output to layer 3. continue until the output layer neuron calculates the output. The result is the output from this ANN…. but at the moment it does not really mean anything.
Back Propagation calculation example
// derivative of the cost/error
dcost_dpred = 2* (output - target);
//bring derivative through sigmoid
dpred_dz = output * (1.0 - output);
// derivative of cost of connected layer
dcost_input1 = dcost_dpred * dpred_dz* my_weight1;
dcost_input2 = dcost_dpred * dpred_dz* my_weight2;
dcost_input3 = dcost_dpred * dpred_dz* my_weight3;
dcost_input4 = dcost_dpred * dpred_dz* my_weight4;
// cost of each input*weight
dcost_dw_1= dcost_dpred*dpred_dz*input1;
dcost_dw_2= dcost_dpred*dpred_dz*input2;
dcost_dw_3= dcost_dpred*dpred_dz*input3;
dcost_dw_4= dcost_dpred*dpred_dz*input4;
dcost_db = dcost_dpred*dpred_dz;
// adjust the weight and bias, calculate how much it needs to adjust
my_weight1 = my_weight1 - dcost_dw_1 * 0.2;
my_weight2 = my_weight2 - dcost_dw_2 * 0.2;
my_weight3 = my_weight3 - dcost_dw_3 * 0.2;
my_weight4 = my_weight4 - dcost_dw_4 * 0.2;
my_bias = my_bias - dcost_db * 0.2;
It takes thousands of cycle to get the weights and bias adjusted for the ANN. Instead of manually doing this cycle, we use python program to calculate the weight/bias adjustment. Here is the result for our network with training data of 0 (open hand) and 1 (peace sign)
----layer 1-------
id: 1
weights: [-0.2507419777064503, 0.6843030508187395, 0.9652874106067459, 1.9210981981244435]
bias: 1.3674968744271552
id: 2
weights: [2.7390957510534855, -1.3541115215534636, -0.745960339661368, 4.6971506183500225]
bias: -2.760673707534653
id: 3
weights: [0.4220142433060409, 1.0191515022947515, 1.3339526130821937, -2.005149331783937]
bias: 0.12055693304754884
id: 4
weights: [1.7794238436106726, -0.13009020832882814, -0.6084139777388159, 2.7603831298267396]
bias: -1.882092139194955
----layer 2-------
id: 1
weights: [0.02261406316791201, -2.433985823319109, 1.6588374988588093, -0.5692445842427973]
bias: 0.09088854764278671
id: 2
weights: [-0.8544765851927711, 4.891256005543523, -0.6468412306798608, 2.253815443973931]
bias: -2.238604005964627
id: 3
weights: [0.3180485620479017, -1.6083379833784954, 0.45504574732365105, -1.5027806486599098]
bias: 0.589103698270661
id: 4
weights: [-0.46327297334421275, -2.3352737931137937, 1.9186227985278832, -0.7831669441448219]
bias: 0.489094090812013
----layer 3-------
id: 1
weights: [1.5048071223386204, -3.737616259602747, 1.5188549954172796, 2.421287474246743]
bias: -0.3622021679730157
id: 2
weights: [-0.8058412774844004, 3.129531561471479, -1.7667000025412236, -1.3133801012031985]
bias: 0.053718164383544084
id: 3
weights: [0.9970237420813575, -0.8943012786359477, 0.35733350276639114, 0.4807725408924337]
bias: 0.6169698994977659
id: 4
weights: [1.9135420836664718, -3.1748064970613536, 1.0751303593104142, 0.30686001360277704]
bias: 0.20865042688502433
----output-------
id: 1
weights: [-5.301352778944949, 3.916295314460912, -0.9226949655532908, -3.3518059869535386]
Now change your weights and bias as above, and let’s try to forward propagate the network with 1 set of sensor data. Does it work now?
Try ml.ann with PD
ml-lib consists many types of machine learning algorithms. The one that use Artificial Neural Network is called “ml.ann”. Make a new object, type in ml.ann. The object by default takes classification model (that tells if it is class 1 or class2). We would liek to use it as Regression model (it shows the range, respect to the training target data you provide). To change this, send message “mode 1”.
To know what options are available and what is the default setting, send message “help” and it will print out all the details in PD window.
To add training data, create message box starting with “add” then the target value (what you want to see in output from ml.ann), then input data set. In this example, the target value is either 1 or 2. when the input is 0.1 0.2 0.3, it should output 1 (or similar) and when the input is 0.6 0.5 0.4, it should output 2 (or similar)
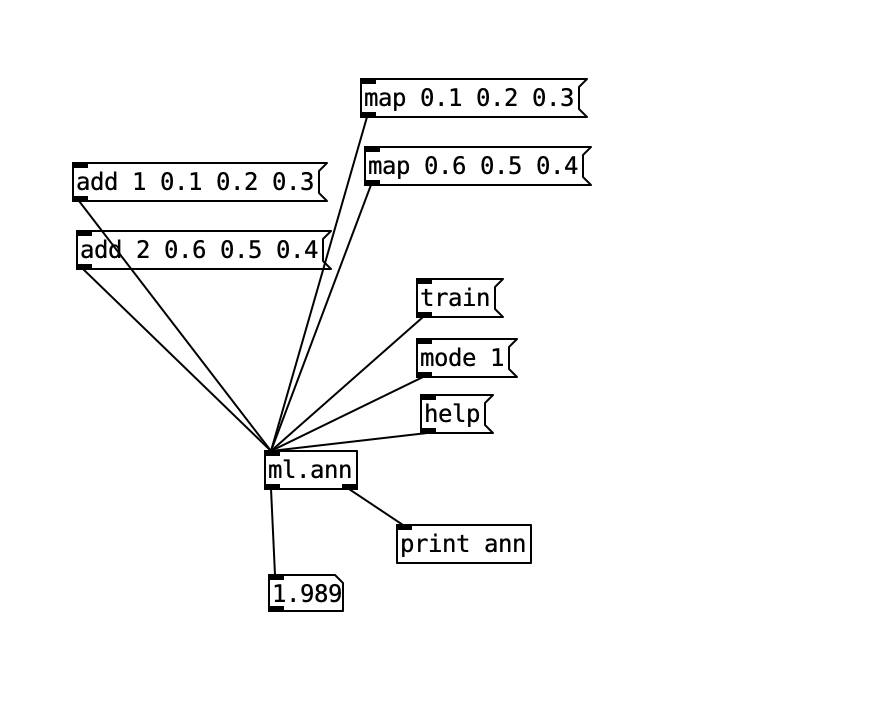
Now, if you map even data set that is not what you trained with like 0.05 0.2 0.3 or 0.1 0.2 0.2, it still gives relatively closed to the target value you trained (0.778 or 0.919). If you give more example set for training, the precise it gets.
You can change the settings, like number of outputs or activation function to see what kind of different result you can get.
For classification, you can use ml.ann, or you can use ml.svm object.
Day 2:
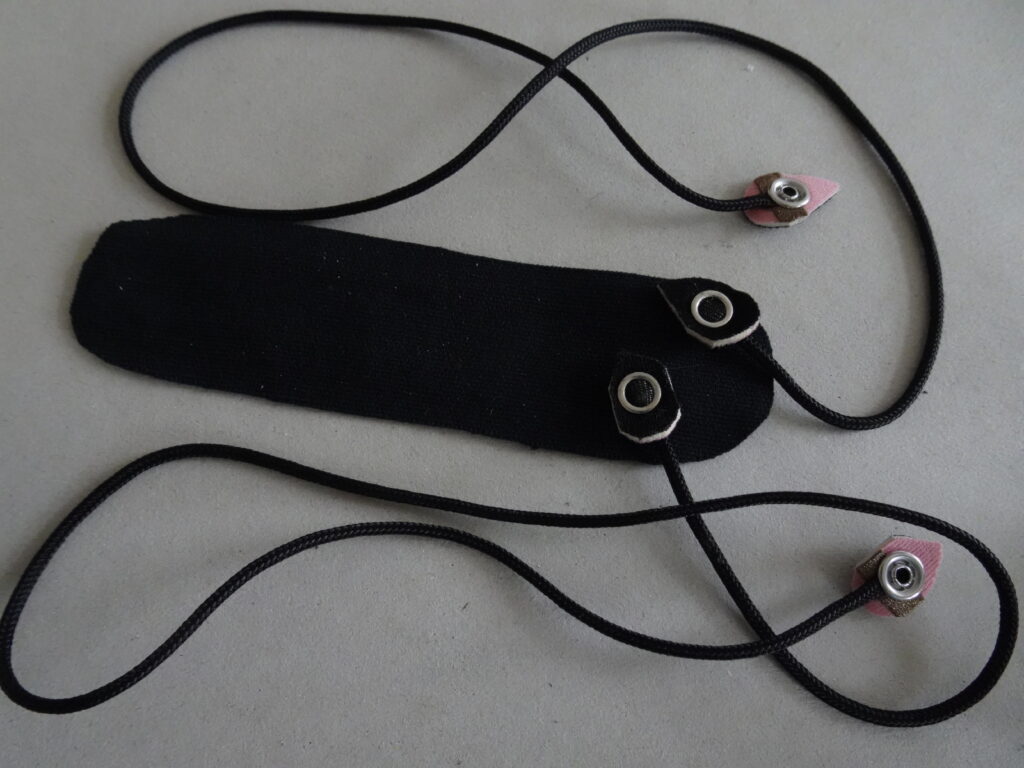
Today, we start with making sensor. Please check your kit. it should contain:
- Teensy LC
- breadboard
- crocodile clip and jumper cables
- resister set
- silver stretch conductive fabric
- eeonyx stretch resistive fabric
- copper thread
- paracord cable x 2
- dress pins x 12
- 1x needle
- kinesiology tape
The detail of how to make sensor is here >> https://www.kobakant.at/DIY/?p=8497
To read the sensor with Arduino/Teensy, you will need to make voltage divider circuit on your breadbaord and read with AnalogRead(). The details of voltage divider is posted here >> https://www.kobakant.at/DIY/?p=8649 https://www.kobakant.at/DIY/?p=6102
To use Teensy, you will need to install Teensyduino >> https://www.pjrc.com/teensy/td_download.html
After you successfully read data with Teensy and monitor through serial monitor or serial plotter, you can also connect PD to read the data in PD. Below is an example of how you can communicate over serial object and purse data (that is separated with space, end with return) in PD.
schematic image of what we were doing this morning with voltage divider
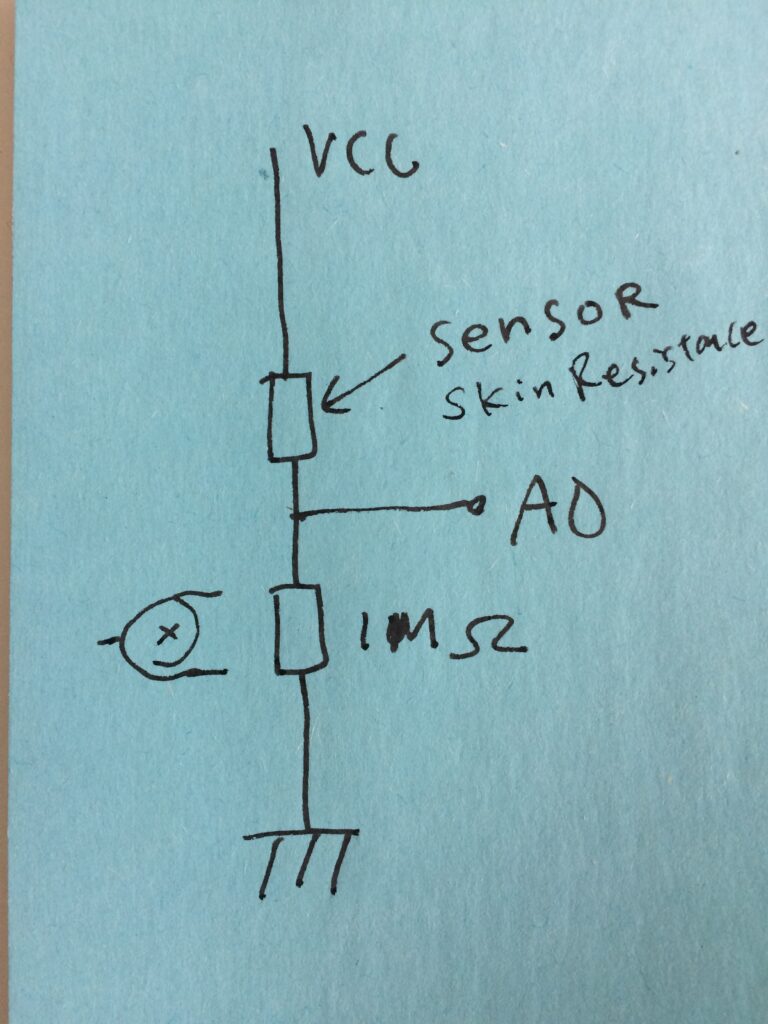
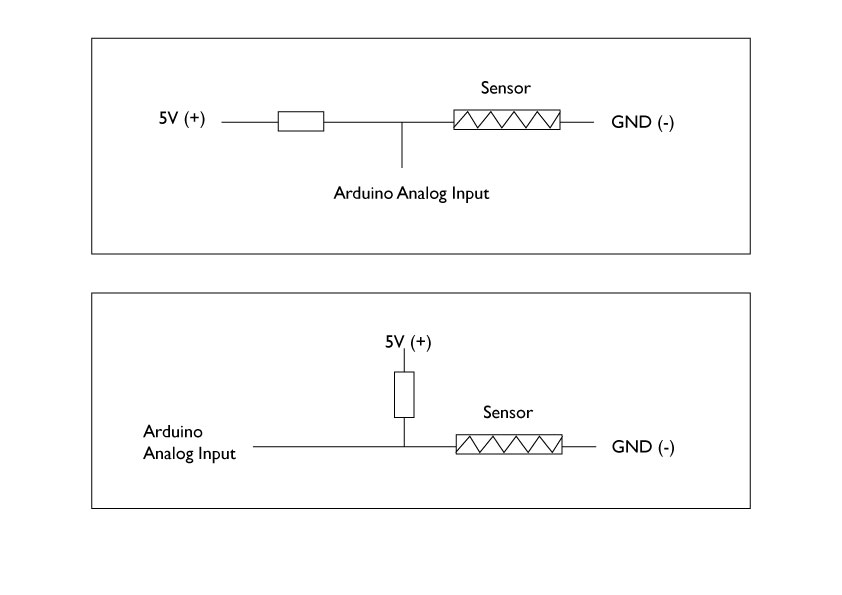
Note that VCC or 5V is depending on the reference voltage of your microcontroler. If you are using Arduino Uno or Mega, it is 5V, if you are using Teensy, it is 3.3V.
Additional external tutorials online
If you are interested in getting into Pure Data, Here is a very nice tutorial series. >> https://www.youtube.com/playlist?list=PLqJgTfn3kSMW3AAAl2liJRKd-7DhZwLlq
If you are not familiar with AnalogRead and voltage divider, there are also a lot of tutorial videos on youtube like this one >> https://www.youtube.com/watch?v=5TitZmA66bI
Floss Manual has also very nice complete tutorial on Pure Data >> http://write.flossmanuals.net/pure-data/introduction2/
There are really many tutorial videos online. Please find the one which works for you, and acquire knowledge you want!
Day 3:
Applying Machine Learning to Kinesiology tape sensor input
This is the final and important step. after successfully reading your sensor data into PD, you can start using them as a set of data to train ML. You can assign multiple sensor data position to a specific parameter for the synthesizer. For example, you can assign a pose or hand gesture to one setting of a synthesizer, and another pose or gesture to another setting. After the training, you will see the ML understanding two poses you have trained, and interpolating the between movements for the parameter.
1: place multiple kinesiology sensor on your body
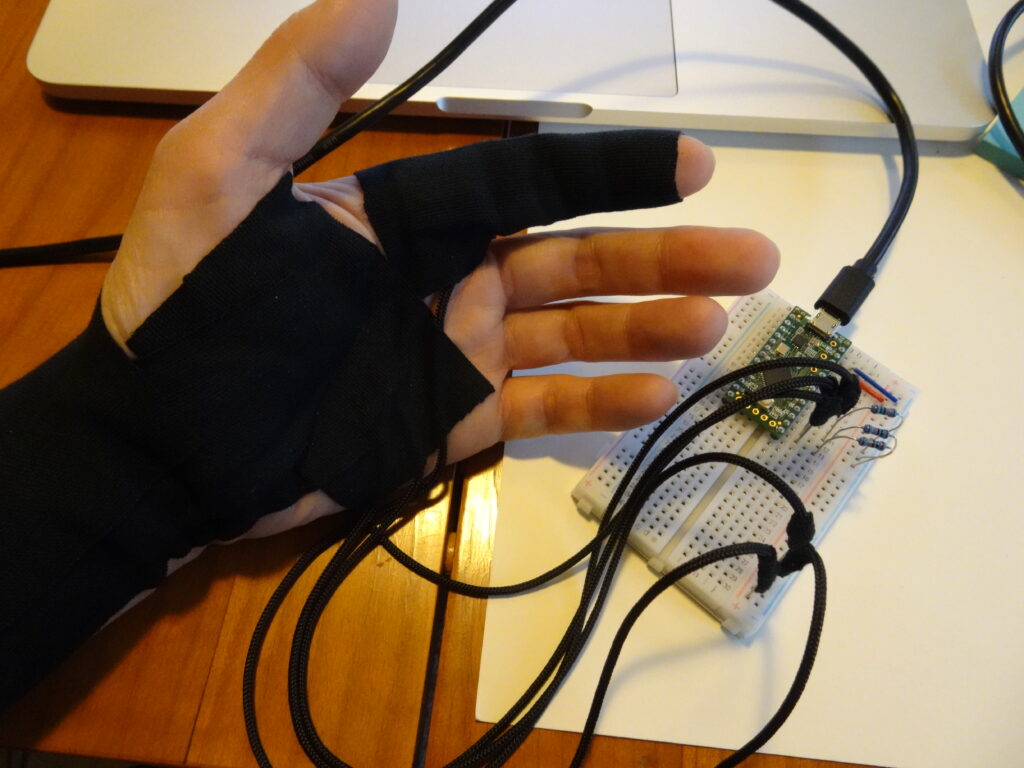
Think about what kind of movement/ interaction you want to capture. Observe the movement and plan where you can place the sensors. Use extra Kinesiology tape to place sensors on body by taping directly on your skin, or taping on top of your cloths items. If you want to capture subtle movement, you can also use cosmetic glue for fake eyelashes to glue the sensor directly on your skin
2: read sensors with Arduino, check with Serial Plotter if you have a good range of reading
Connect sensors with Arduino. You will need voltage divider for each sensors.
Then you can program Arduino to read Analog pins you have connected the sensors with, and print the value to serial communication. When you have multiple sensors connected, separate the values with space ” ” so later on your PD patch can parse the data.
You can alternatively use the example sketch on DEMO folder on the course material. Here is the same code on the github >> https://github.com/mikst/IC_workshop/tree/master/DEMO/analogRead_6sensors
You can open Serial Plotter on Arduino IDE to check your sensor readings. You can add 0 and 1023 in the printing chain to fix the range for plotter. If your reading range is not very big, you can also switch the value of your voltage divider resisters. With eeonyx resistive fabric constructed in the example way shown in kinesiology tape sensor post, it will measure somewhere around 200k ohm to 10k ohm, so your voltage divider could be 100 k ohm or 47 k ohm or 10 k ohm. Find the resister that works the best with your sensors.
3: read sensor data from PD
Close Serial Plotter or Serial Monitor on your Arduino. Open Pure Data patch serial_communication_example.pd from the DEMO folder. >>https://github.com/mikst/IC_workshop/blob/master/DEMO/serial_communication_example.pd
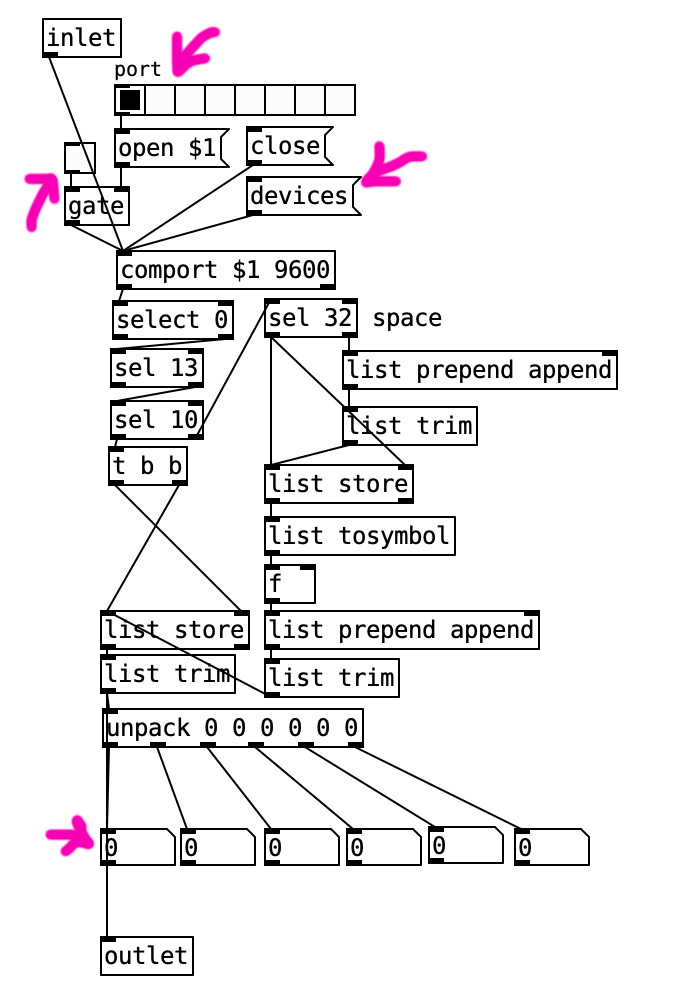
click devices to see which port your Arduino is connected. You will see the list of devices in your PD window.
Then open the gate (you see X in the box) and choose the port you want to open. The radio button starts with 0. (0,1,2,3,….)
When you open the port and start getting your sensor data, you will see the receiving number in the number box in the bottom. The example is made to read 6 sensors. So if you’ve connected only 3 sensors, the first 3 is your sensor data, and the rest is just a noise. You can ignore them or erase the number box.
4: try connecting data directly with synthesizer control
Open sound_synth_ example.pd from the DEMO folder >> https://github.com/mikst/IC_workshop/blob/master/DEMO/sound_synth_%20example.pd
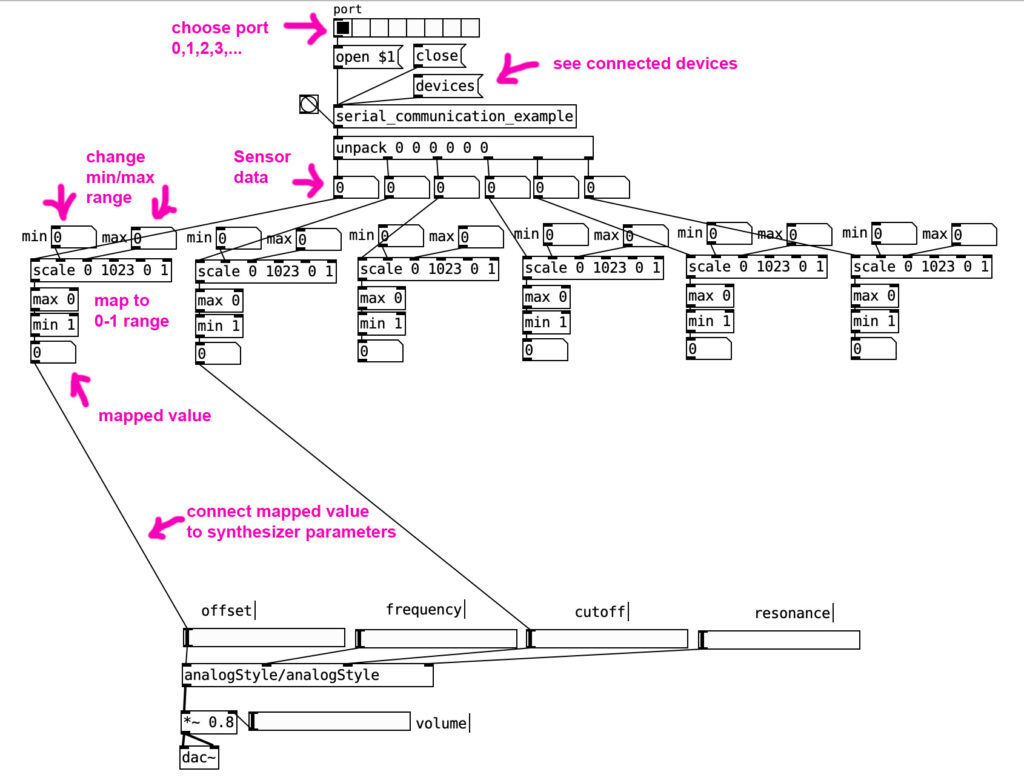
Open the port connected to Arduino and you will see the sensor data coming in. The synthesizer parameters are set as 0-1 range. So, you can scale/map the sensor data (0-1023) to the parameter range (0-1) using scale object. Often textile sensors does not use the whole range of 0-1023. You can observe the min/max of your sensor reading range and manipulate the min/max of the scale object. You can do this either by sliding the number box connected to the 2nd and 3rd input of scale object or directly change(type in) the first 2 arguments on the scale object. max and min object after the scale object is constraining the result from the scale object to 0-1 range. You will see the result of the range scaling at the number box connected to it. You can connect this mapped value to any of the synthesizer controlling parameter on the bottom and see what kind of sound effect you can achieve.
5: try using ml-lib. Train with your sensor data and map
Open ANN_synth_example.pd from Demo folder. Or it is also posted on github >> https://github.com/mikst/IC_workshop/blob/master/DEMO/ANN_synth_example.pd
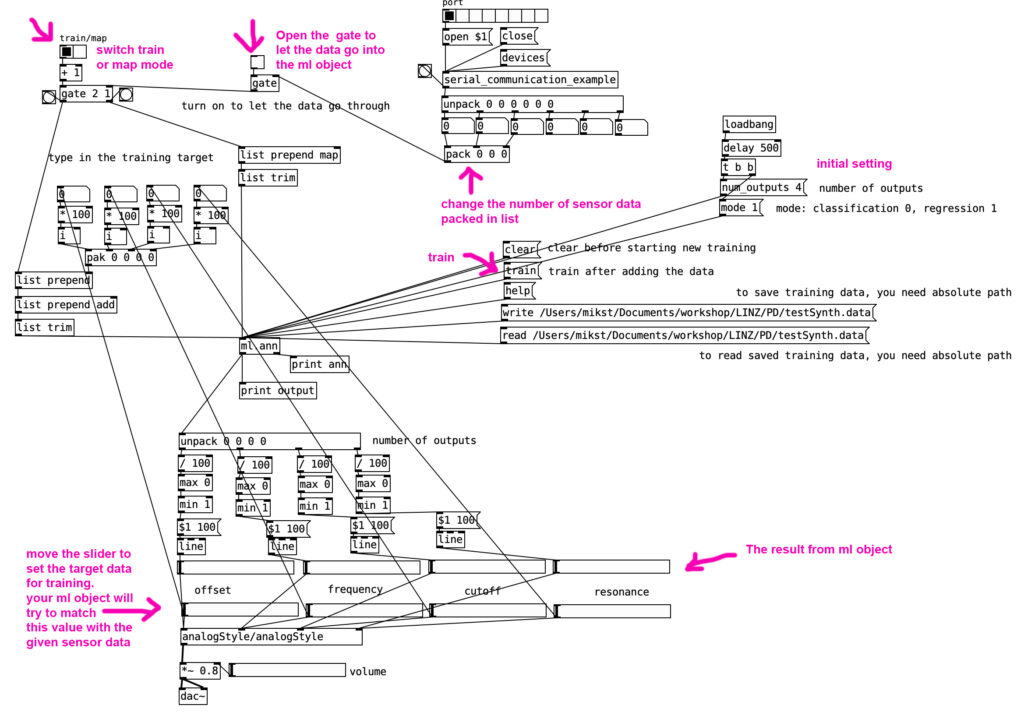
Open the port and read your sensor data in. adjust the number going into the pack object. For example, if you are using 4 sensors, edit the “pack 0 0 0” to “pack 0 0 0 0”. You will see one more inlet on the top if the object. Connect the fourth number box to the 4th inlet.
Make sure that your mode switch is on “train” side. Move around and decide few poses you want to train. You can train as many poses as you like.
Adjust the 4 sliders (offset, frequency, cutoff, resonance) and set the target control data for your training. Once you have a good control slider setting, fix your body posture to the first pose you want to train, then open the gate located on the top center of the patch and let your sensor data go into your ml object for few seconds. The Close the gate and you can move again.
Now move the sliders to next setting, move to the second pose and open the gate on the top again for few seconds. Close the gate and relax.
Continue the process for all the poses you want to train.
After you finish adding all the training data, you can click “train” message object. In the PD window, you will see “ann: train 1” printed out. This means your ml.ann object is trained to the data you gave.
Switch the mode to map (top left corner) and open the gate. Now your sensor data is going into ml object to map with your training model. It gives out 4 output values that corresponds to the training data you gave. move around and try to do the example pose you gave. Does the sound/parameter match with what you gave as example?
Once you understand how ml object and training works, you can try different variations of training. Before starting new training, click clear message box above training message box to clear the ml object.
Leave a comment